Data Lakehouse Explained
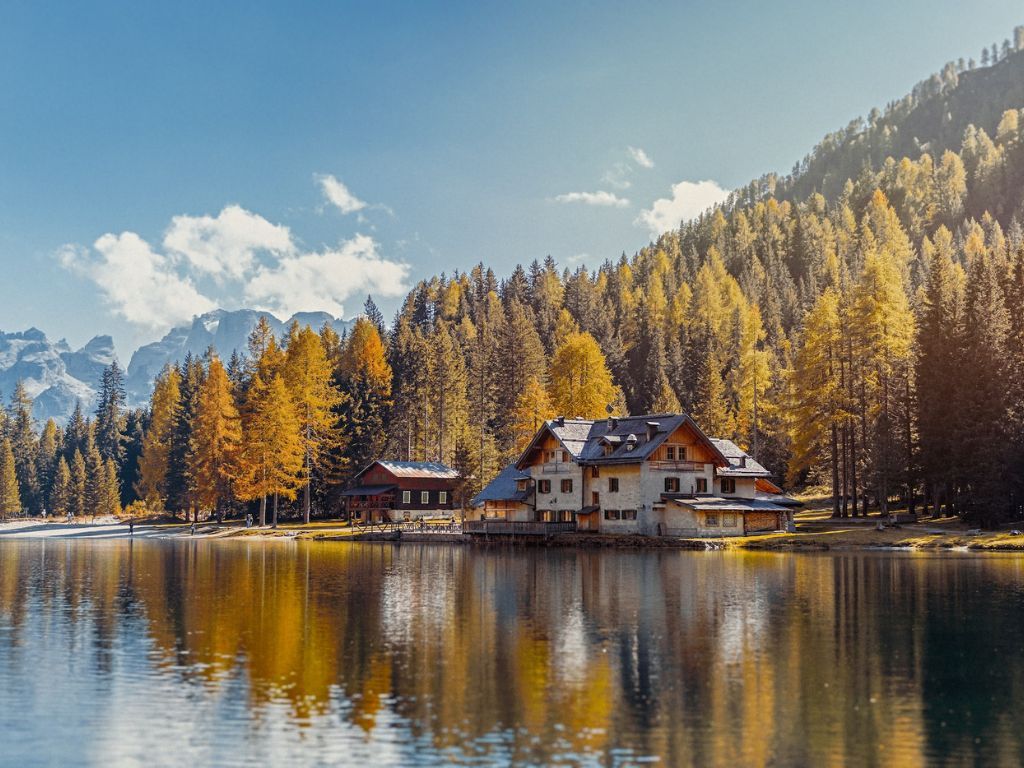
Occasionally, I find myself craving for chimaek, the perfect combo of Korean fried chicken and beer. This flavourful union of crispy chicken and bubbly beer is nothing short of heavenly. Just last night, I succumbed to my cravings that had been simmering for weeks.
We were a group of five, and we devoured the fried chickens, needing to order seconds because it was simply too tasty! 😋
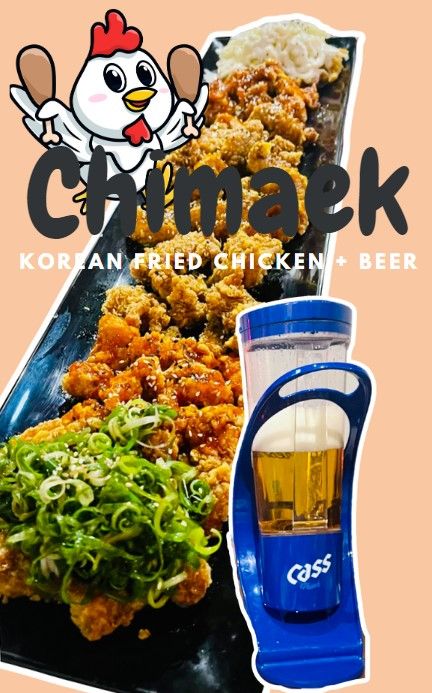
You might be wondering, what does this have to do with data lakehouses? Interestingly, the process of a restaurant transforming raw ingredients into these crunchy, succulent fried chickens can be compared to the operation of a data lakehouse.
In this blog, we'll delve into the concept of data lakehouses and their architecture. This term came into being around 2010 and rapidly gained widespread adoption. A data lakehouse is an approach that amalgamates the strengths of data lakes and data warehouses. I'll break down these terms for you shortly.
The Concept of Data Lakehouse
Imagine a bustling commercial kitchen. We have raw ingredients delivered by trucks and stacked on large pallets at the loading dock. These ingredients must then be unwrapped, sorted, labelled, and stashed in the appropriate areas - a pantry for dry goods or refrigerators/freezers for perishables. The systematic organisation and correct handling of these ingredients are essential to maintaining food safety and minimising waste. Without an efficient system, chefs would struggle to perform their tasks effectively.
The realm of data can be as chaotic as a hectic restaurant kitchen. Much like how ingredients are delivered to the loading dock, many data types pour into organisations from various sources, such as cloud environments, operational apps, and social media platforms.
Amid this data chaos, data lakes can be considered the landing site for all types of data - raw, structured, unstructured, and semi-structured. If you're unclear about the difference between these data types, refer to one of my previous blogs on this topic.
Like how we can't cook on the loading dock, we can't extract insights directly from a data lake. The data needs to be sorted and transformed, akin to processing raw ingredients in the kitchen and stored in data warehouses.
We can think of data warehouses as the kitchen's pantry, fridges, and freezers, which optimise everything for specific data tasks. They power activities such as building dashboards, creating reports and supporting other analytics tools. Much like how a kitchen maintains its pantry, data in the warehouse is properly managed and governed, ensuring its reliability.
Data lakes and warehouses each have their own advantages, but they also have their limitations. Data lakes run the risk of devolving into "data swamps," cluttered with duplicated, incorrect, or incomplete data. On the other hand, data warehouses can be costly and sluggish for certain data types.
Here's where a data lakehouse comes into play. It embodies the best features of data warehouses and data lakes, combining a lake's cost-effectiveness and flexibility with a warehouse's structured performance.
A data lakehouse allows organisations to store, manage, and use data from various sources without overspending. It also comes equipped with management governance tools necessary to supercharge business intelligence and power rapid machine learning workloads.
But how exactly does a lakehouse resolve the issues inherent in data lakes and warehouses? A data lakehouse effectively tackles the issues inherent in data lakes and warehouses.
Firstly, it mitigates data duplication. When a company uses multiple data warehouses and a data lake, it often leads to data redundancy - the same data piece stored in multiple locations, causing inefficiency and potential inconsistency. A data lakehouse consolidates everything, eliminates additional data copies, and establishes a single version of truth for the company.
Additionally, a data lakehouse bridges the gap between analytics and business intelligence (BI). Data scientists often use analytics techniques on data lakes, while BI analysts use a data warehouse. A data lakehouse facilitates both teams to work within a single shared repository, thereby reducing data silos. It also addresses data staleness by supporting real-time streaming (continuous data flow) and micro-batching (processing data in small batches), ensuring analysts have access to the most recent data.
By integrating the functions of a data warehouse into data lake technology, a data lakehouse significantly enhances efficiency.
Data Lakehouse Architecture
To some, the data lakehouse concept might still seem a bit abstract. It was for me when I first heard about it. What helped me understand it better was a diagram of its architecture, similar to the below image. A data lakehouse typically comprises five layers: ingestion, storage, metadata, API and consumption. Lets take a look at layer.
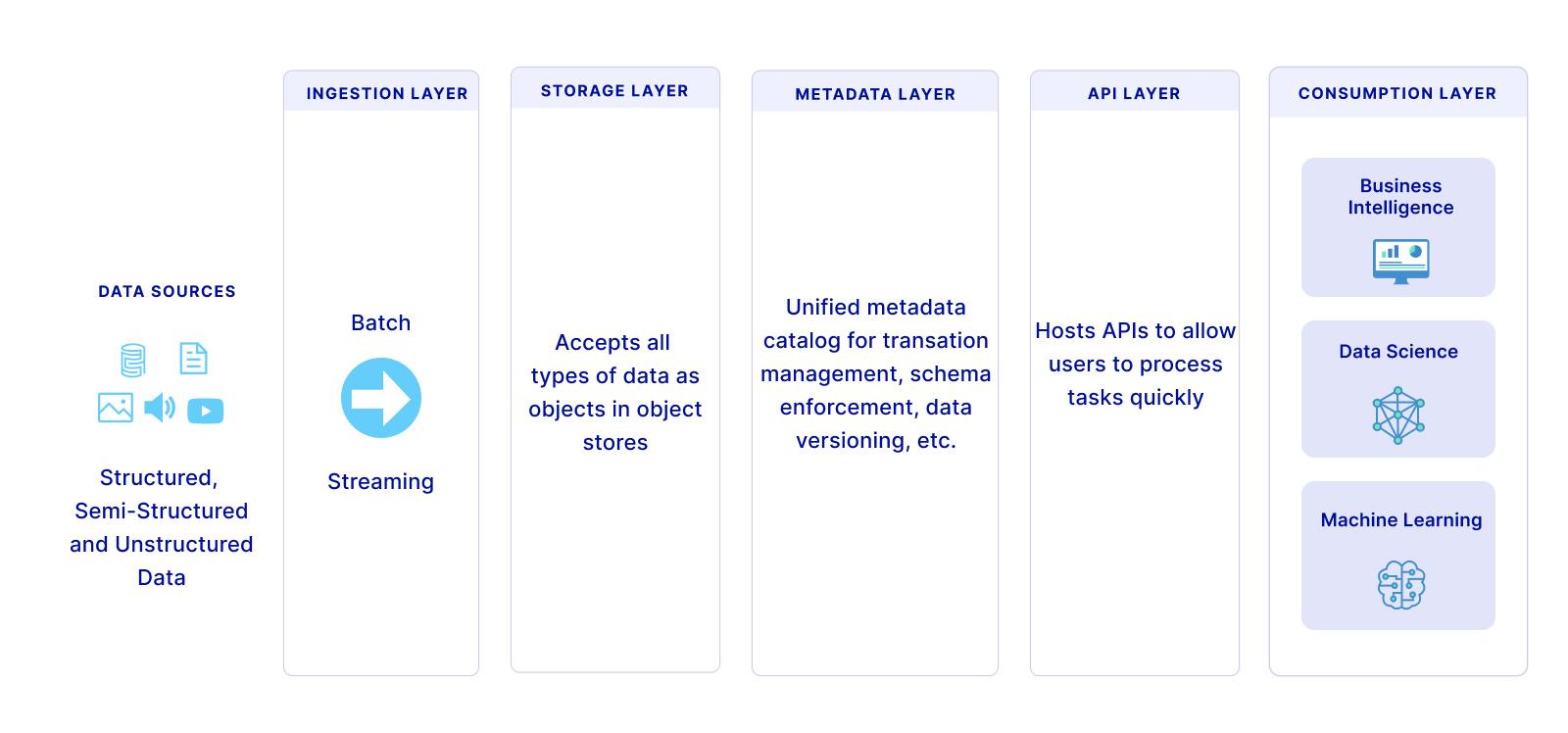
1. Ingestion Layer:
This is the data collection layer and acts like a big net, pulling in data from different places like customer management systems or different types of databases. It sends this data to the next level.
2. Storage Layer:
This is where all the collected data is stored. It accepts all kinds of data - like neatly organised (structured), partially organised (semi-structured), or messy (unstructured) data - and keeps them safe in cost-effective storage spaces, such as Amazon's S3.
3. Metadata Layer:
This can be thought of as the organisation layer. This layer is like a librarian for your data. It keeps track of what data you have, where it's stored, and how it's formatted. It lets you do things like creating tables, and updating or inserting data, making everything work smoother and faster. This is the warehousing layer.
4. Application Programming Interface (API) Layer:
This is the connection layer. It helps users and developers get to the data they need quickly. It's like a translator, allowing different programming languages and tools to understand and work with the data stored in the lakehouse.
5. Consumption Layer:
This is the layer where people in the company get to use the data. It hosts tools like Tableau, allowing everyone to access the data they need for things like creating reports and dashboards or visualising the data in different ways.
While a data lakehouse isn't a magical solution for all data-related challenges, there are many scenarios where it can add value. For instance, if you aim to use both artificial intelligence and business intelligence, a data lakehouse could become an integral part of your toolkit. It can also address data inconsistencies and redundancies that crop up when dealing with numerous systems.
Consider this- in a busy restaurant kitchen, the chef must have all ingredients, tools, and recipes at hand to craft a delightful dish. Similarly, a data lakehouse serves as a one-stop shop for your data needs, efficiently gathering, storing, and analysing data to deliver valuable insights for your business. Next time you're relishing a meal at your favourite restaurant, think about the artistry behind the scenes - it's a similar magic in the world of data!