Frequentist vs Bayesian Statistics: Understanding the Key Differences
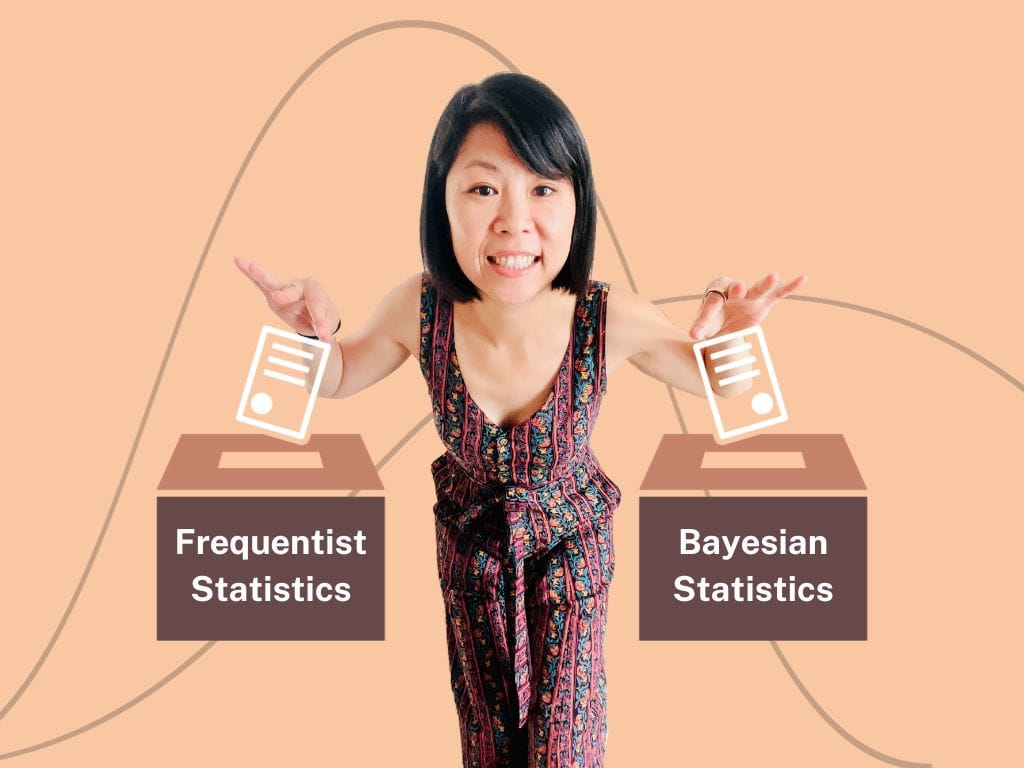
Hi everyone! I'm back from my holiday. Did you miss me? It's been hard to get back into the swing of things, but I think I'll manage.
Before I departed for my holiday, many of my blogs have been on exploring statistics. It's important in big data and data science and other fields like health economics, which is relevant to many healthcare systems, including Australia. We're pretty lucky in Australia to have government-subsidized healthcare. The Pharmaceutical Benefits Scheme (PBS) is a big part of this, which helps make many prescription drugs more affordable. Working in a pharmaceutical company, I've been exposed to how the government and drug companies negotiate prices and the intensive stats work that goes into evaluating a drug for PBS listing. It's all about weighing the cost of treatment against the health benefits it brings.
Why am I talking about this? A former colleague from the Market Access department (they handle getting new meds onto the PBS) asked for a piece on Frequentist vs Bayesian Statistics. I thought it'd be a great idea to share this with all of you.
In this blog, we'll cover:
• What Frequentist and Bayesian Statistics are
• Pros and Cons
• How to Choose Between Frequentist and Bayesian Statistics
Let's get started!
What Frequentist and Bayesian Statistics are
Frequentist and Bayesian statistics are two distinct approaches to statistical inference, each with a unique philosophy and methodology.
Does the term statistical inference sound foreign to you? Let me explain. Statistics is generally divided into two types: descriptive and inferential.
Descriptive statistics is about summarizing data, like calculating averages or understanding the range of values. It's like a snapshot of what your data is saying at a glance.
Inferential statistics, on the other hand, is about making educated guesses about a larger group from a smaller sample. Imagine asking a few people in a city about their favourite food and predicting the most popular choice citywide.
Now, let's go back to Frequentist and Bayesian statistics. These two terms get thrown around a lot in the statistics community. When I first heard of these, I thought there was some huge war where one side was trying to gain control of all of the statistics, but that's not exactly how it is. Hmmm…. I wonder if this is why my ex-colleague requested that I write about this topic.
It's not about one being better than the other; most people find themselves somewhere in between.
We won't dive deep into the math but focus on their philosophical differences. To grasp the core differences between Frequentist and Bayesian statistics, it's essential to understand how each defines probability.
Bayesian statistics, however, combines the results of events with personal beliefs or prior knowledge. Grounded in Bayes Theorem (more on this in my Bayes Theorem Blog), it initially seems counterintuitive as it allows for different answers based on individual beliefs. Back to the coin example: a Bayesian might assign a probability to heads based on prior experience, even before flipping the coin. For example, they might say there's a 50% chance of heads because, in their experience, coins are usually fair. Another Bayesian might assign a different probability, like 25%, based on their experiences, and both could be considered correct.
This distinction highlights how Frequentists rely purely on empirical data, while Bayesians incorporate prior knowledge into their probability calculations, updating their beliefs with new data.
Another key aspect of Bayesian statistics is the concept of updating beliefs with new data. In Bayesian philosophy, your initial probability estimate is not set in stone. For instance, if you initially think a coin has a 50% chance of landing heads, but someone else believes it's 25%, both of you would start updating your beliefs as you collect more data from flipping the coin. As the number of flips grows, your beliefs gradually align more closely with the actual data observed. Eventually, with enough data, your initial beliefs become less significant, and the probability you assign to the coin landing heads is primarily based on the observed outcomes.
In contrast, Frequentists argue against the idea of prior beliefs. They believe in a definitive probability that exists irrespective of personal belief, which can only be determined through data collection. So, a Frequentist would start collecting data right away to discover the true probability, without any preconceived notions about what it should be. This difference in approach highlights how Bayesian statistics is more about a flexible philosophy of thinking, integrating both prior beliefs and real-world data, whereas Frequentist statistics strictly adhere to empirical data for making statistical inferences.
Pros and Cons
So, what are the pros and cons of the Frequentist and Bayesian statistics approaches?
Pros of Frequentist Statistics
-
Objective Results: Frequentist methods give clear-cut results based on the data, which is good for situations like clinical trials where you want no-nonsense answers.
-
Widespread Adoption: Frequentist methods are widely accepted and used across various fields, like finance, healthcare, engineering etc., offering a common and reliable framework for statistical analysis.
-
Clear Interpretation: Results from frequentist analyses typically have straightforward interpretations, making them accessible to a broad audience. For instance, frequentist statistics can provide clear insights into public sentiment when analysing opinion poll data.
Cons of Frequentist Statistics
-
Limited Handling of Subjectivity: Frequentist statistics struggle to incorporate subjective beliefs or prior knowledge. This limitation can lead to incomplete assessments, especially when such information is valuable. For example, when assessing the potential success of a marketing campaign without considering market experts' insights.
-
Requires Large Sample Sizes: Frequentist methods may yield unreliable results when working with small data sets. This limitation can restrict their usefulness in cases where data collection is challenging, such as when studying rare diseases or conducting experiments with limited resources.
-
Lack of Adaptability: Frequentist techniques do not easily adapt to changing beliefs or rapidly evolving data. This inflexibility can make them less suitable for dynamic environments, like financial markets, where conditions change rapidly.
Pros of Bayesian Statistics
-
Incorporates Subjectivity: Bayesian statistics embrace subjective beliefs and prior knowledge, offering a holistic approach to decision-making. This makes them suitable for scenarios where expert insights or personal opinions, such as investment portfolio management, play a significant role.
-
Flexibility with Small Data: Bayesian methods can provide meaningful results even when working with limited data, making them valuable when data collection is constrained. For instance, Bayesian analysis can tailor treatments for rare diseases with few patient records in personalised medicine.
-
Continuous Learning: Bayesian can continuously update their beliefs as new data becomes available, allowing for adaptability and improved decision-making over time. Bayesian models can adjust to changing circumstances and evolving data streams in fields like machine learning.
Cons of Bayesian Statistics
-
Complexity: Bayesian analysis can be more complex, requiring careful consideration of prior beliefs. This complexity can introduce subjectivity into the analysis, which must be managed effectively. For example, estimating the probability of success for a new business venture may involve complex Bayesian modelling that incorporates various subjective factors.
-
Computationally Intensive: Bayesian calculations may demand significant computational resources, which could be a limitation in resource-constrained environments. For instance, in climate modelling, Bayesian techniques may require substantial computing power to simulate complex climate systems accurately.
-
Less Commonly Used: Bayesian statistics are less prevalent in some fields, which can present challenges in communication and adoption, particularly when introducing Bayesian approaches to stakeholders who are more familiar with frequentist methods.
How to Choose Between Frequentist and Bayesian Statistics
The decision between frequentist and Bayesian statistics isn't one-size-fits-all. It hinges on factors like the nature of your data, research goals, and the presence of prior information. Here's what I've learned during my research:
When to Use Frequentist Statistics
-
For Objectivity: If you need results that are unbiased and untouched by personal opinions, frequentist statistics are your go-to. They excel in scenarios where impartiality is important, like scientific studies, clinical trials, or quality control processes.
-
With Large Data: Frequentist methods shine with ample data. If you have a substantial dataset at your disposal, frequentist statistics can provide dependable and stable estimates.
-
In Data Collection Challenges: When gathering data is tough or costly, frequentist methods come to the rescue. They don't rely on prior beliefs, making them suitable for situations where you have limited prior information.
-
For Well-Established Procedures: Frequentist techniques come with tried-and-true procedures and formulas, making them a trusted choice for common statistical analyses.
When to Choose Bayesian Statistics
-
Incorporating Subjectivity: Bayesian statistics welcome subjective beliefs and prior knowledge. This flexibility is valuable when dealing with complex or uncertain situations and allows you to integrate expert insights into your analysis.
-
With Small Data: Bayesian methods can yield meaningful results even with limited data, making them a lifeline when data is scarce or when you're exploring rare events.
-
For Continuous Learning: When your analysis needs to evolve with fresh data, Bayesian statistics are a match. They permit iterative updates to beliefs as new information surfaces, making them invaluable in dynamic environments.
-
Customised Decision-Making: Bayesian approaches offer the flexibility to tailor results based on individual preferences or specific circumstances, facilitating customised decision-making.
What I gathered is, in reality, choosing between Bayesian and Frequentist methods isn't a strict either-or decision. Many researchers blend the two, tapping into Bayesian strategies for integrating prior beliefs and Frequentist techniques for objective hypothesis testing. The key is to match the approach with the research goals, the nature of the data, and the philosophical angle that best fits the needs.
So there you have it. I hope this sheds some light on the differences between these two approaches and shows you that they're not mutually exclusive. It's all about knowing when to use one, the other, or a mix of both to get the best results for the project.