Intro to Customer Segmentation & RFM Analysis
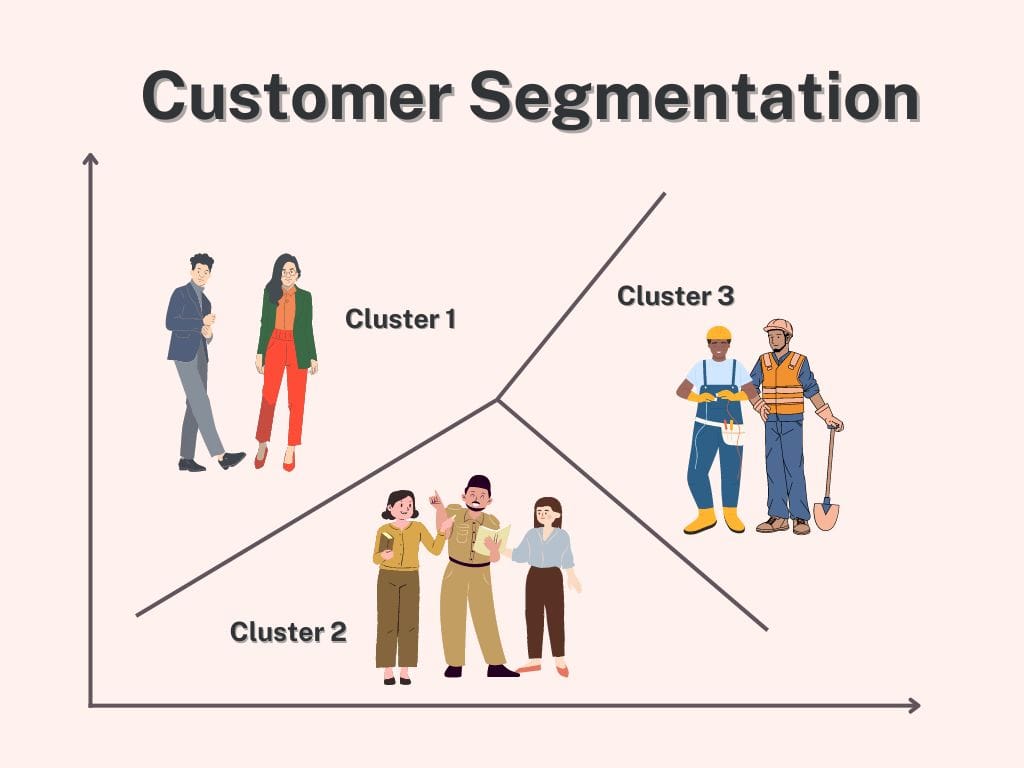
I remember the first time I participated in a segmentation exercise. It involved sending Excel files to sales representatives to categorise their customers into segments A, B, C, and D. The files often came back messy, leading to many back-and-forths with the field teams. It was time-consuming and laborious, and the work wasn't fascinating. I wondered: Is there another way to do segmentation, and can it be done more quickly? The answer to both is yes!
You’ve guessed it! We’re going to look at customer segmentation. We will look at:
- What is Customer Segmentation?
- How is it Different from Cohort Analysis?
- Four Common Types of Market Segmentation
- RFM Analysis
What is Customer Segmentation?
Customer segmentation is a technique to divide your customers into subgroups based on shared characteristics like age, income, location, etc. Why do this? It enables you to tailor your marketing efforts to your ideal customer profile.
For example, a customer who shops at an organic food store will likely have a high salary, be health-conscious, and be interested in healthy eating and exercise. Instead of wasting the budget on broad campaigns, focus marketing efforts on campaigns that speak directly to the target market segment.
How is it Different from Cohort Analysis?
I wrote a blog on Cohort analysis; if you’ve missed it, check it out here: Cohort Analysis- What is it? What do you do with it? How to read it?
Cohort analysis and customer segmentation are methods used to understand customer behaviour but differ in focus and approach. Cohort analysis is time-based and groups customers by shared characteristics during a specific period (e.g., customers who signed up in January). It helps understand how these groups behave over time, such as tracking retention or purchasing patterns. In contrast, customer segmentation aims to target defined customer segments better.
Four Common Types of Customer Segmentation
There are four main types of customer segmentation:
1) Demographic Segmentation: Dividing the market based on demographic information such as age, gender, income, education, occupation, marital status, and family size.
2) Geographic Segmentation: Customers are categorised based on their geographic location, such as country, region, city, or postal code. People in the same geographic region typically have similar needs, mindsets, and cultural preferences. This segmentation provides insight into geo-specific variables like climate, culture, language, and population density.
3) Psychographic Segmentation: Grouping customers according to their lifestyle, values, interests, attitudes, and personality traits. This segmentation focuses on the psychological aspects of customer behaviour. Although it is more difficult to gather data for, however, social media is a good source. Other ways to collect this information are through surveys, interviews and focus groups.
4) Behavioral Segmentation: Dividing the market based on customer behaviour, including purchase patterns, usage rate, brand loyalty, benefits sought, and readiness to buy. This segmentation helps understand how customers interact with products and services. A typical analysis associated with behavioural segmentation is RFM analysis.
RFM Analysis
In this section, I’ll explain RMF analysis and the high-level steps in conducting this form of analysis manually and using machine learning.
What is RFM analysis?
It is a form of behaviour segmentation analysis that’s used to understand customer purchasing behaviour by looking at these three factors:
Recency (R): How recently a customer made a purchase. Recent buyers are more likely to respond to marketing efforts.
Frequency (F): How often a customer makes a purchase. Frequent buyers are usually more loyal.
Monetary Value (M): How much money a customer spends. High spenders are often the most valuable.
By scoring customers on each of these dimensions, businesses can segment their customers into easier target groups with tailored marketing strategies. For example, a company might identify a segment of high-value, frequent customers who have not made a recent purchase and target them with a special promotion to encourage re-engagement.
Steps Involved in RFM Analysis
You can do RFM Analysis with or without machine learning. I recently learned how to do it using machine learning, specifically K-Means Clustering, and it’s much faster! For those unfamiliar with K-Means clustering, it is an unsupervised machine-learning algorithm that groups data points into a predefined number of clusters (K).
I’ve always hated tedious manual work, so I’m a big fan of automation and efficiency. Here’s a comparison of the manual and machine learning methods for RFM Analysis. While it may not look like a significant time saver based on the table output, trust me, machine learning is much faster!
Steps | Manual Process | Machine Learning Process |
---|---|---|
Data Collection | Gather transaction data such as customer ID, purchase dates, frequency, and amount spent. | Gather transaction data such as customer ID, purchase dates, frequency, and amount spent. |
Data Preprocessing | Clean data, calculate RFM Metrics: Recency, Frequency, Monetary Value. | Clean data, calculate RFM Metrics: Recency, Frequency, Monetary Value. |
Feature Engineering | Create R, F, M features for each customer. | Create R, F, M features for each customer. |
Feature Scaling | Not required. | Normalize or standardize RFM features to ensure they are on a similar scale. |
Scoring/Clustering | Assign scores to R, F, M metrics manually (e.g., using quintiles). | Use clustering algorithms like K-Means to segment customers. |
Combine Scores | Combine R, F, M scores to create an overall RFM score. | No need to combine manually; clustering algorithm groups similar customers. |
Segment Customers | Group customers based on combined RFM scores into segments like Champions, Loyal Customers, At Risk, etc. | Analyze clustering results to identify customer segments. |
Cluster Analysis | Analyze the characteristics of each RFM segment. | Analyze the characteristics of each cluster to understand customer types. |
Note that you’ll need to use techniques such as the Elbow Criterion to determine the optimal number of clusters for the model.
So there you have it. Customer segmentation is important and foundational to any successful long-term marketing strategy, and RFM is a very useful analysis to uncover subtle patterns in customer data. I hope you picked up some nuggets in this post. Until next time, ciao!