Statistical Sampling- An Overview
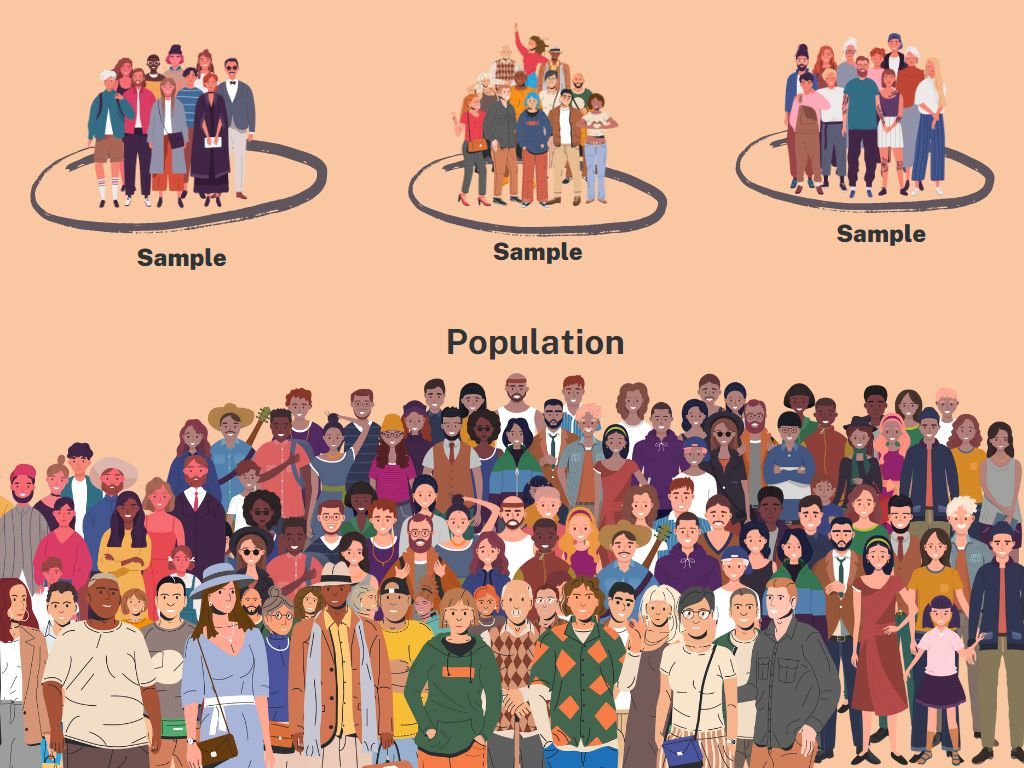
I've sat in meetings where market research firms or business experts confidently share their findings from market research studies. As they assuredly present their conclusions and generalize them across a broad population, I can't help but feel a touch of unease. Silently, I can't help but question whether it is genuine confidence or a blind spot allowing them to draw such clear-cut conclusions from a small sample size, possibly tainted with bias.
Many of these findings are then used for decision-making, and it's concerning. So in this blog, I want to look at sampling. I think it's an important topic that doesn't get enough attention. I believe a solid understanding of sampling is necessary because it enables one to assess the outcome's reliability, validity and generality. This topic isn't just relevant to market research but applicable to all statistical studies, of which market research is one of many. Other examples of statistical studies are A/B testing, social science research, quality control etc.
The areas we will cover in this blog are:
- What is sampling, and Why is it Vital?
- Types of Sampling Methods
- Determining Sample Size
What is Sampling, and Why is it Vital?
We often want to get some information about a large group of individuals but are only able to collect information on a small proportion of that group because it is highly impractical or prohibitively expensive. In statistical terminology, the large group is called a population, and the part of the group in which we collect info is called a sample. Sampling is the process of choosing a set of individuals from a larger population to deduce insights about the entire group.
Ideally, we would like our sample to represent the population as closely as possible. Unfortunately, no methods can guarantee that a sample fully represents the population. The best we can do is use a sampling method that makes it very likely that the sample will be similar to the population.
Types of Sampling Methods
There are many sampling methods, some good and some not so good. The method used depends on what you're studying and the resources available.
Here are six sampling methods which we will look at.
- Convenience Sampling
- Voluntary Response Sampling
- Simple Random Sampling
- Stratified Random Sampling
- Cluster Random Sampling
- Systematic Random Sampling
Convenience Sampling
Convenience sampling is where the researcher selects a sample that is conveniently accessible and not chosen randomly.
Imagine you're trying to understand what your city thinks about a new park that's been proposed. But talking to everyone in the city is too time-consuming and expensive. So, you decide to just ask people at the local grocery store because it's convenient for you. That's convenience sampling. It's easy to do, but it might not fully represent the opinions of the entire city because you're only asking people who happen to be at the grocery store.
Voluntary Response Sampling
With voluntary response sampling, the researcher requests members of a population to join the sample, and people decide whether or not to be in the sample.
Here, you let people come to you. Let's say you put out a call on social media asking what people think about a new restaurant. The people who choose to respond are your sample. This is voluntary response sampling. The downside is that people who feel strongly about the topic (either positively or negatively) are more likely to respond, which could skew your results.
Simple Random Sampling
Simple random sampling is where every member and set of members has an equal chance of being included in the sample and requires random number generators or some sort of chance process to get a simple random sample.
Let's assume you've got a list of all the students at a school, and you want to know their opinion on the school food. To do a simple random sampling, you would randomly pick names from that list (perhaps by drawing names out of a hat or using a computer program). Each student has an equal chance of being picked. This method is good because it doesn't favour any group of students.
Stratified Random Sampling
With stratified random sampling, the population is first split into groups. The overall sample consists of some members from every group. The members from each group are chosen randomly.
Here, you first divide your population into different subgroups, or 'strata'. Let's say you want to understand your town's favourite ice cream flavour across different age groups. You might split your population into 'strata' like kids, teenagers, adults, and seniors. Then you take a simple random sample within each group. This way, you ensure that you have a representation from each age group.
Cluster Random Sampling
With cluster random sampling, the population is first split into groups. The overall sample consists of every member from some of the groups. The groups are selected at random.
Suppose you want to survey several schools in your district, but visiting each one is not practical. With cluster sampling, you could randomly select a few schools (the clusters) and then survey all students within those selected schools. It's like throwing a dart at a map of the district, and wherever it lands, you survey all the students in the closest school.
Systematic Random Sampling
In systematic random sampling, members of the population are put in some order. A starting point is selected at random, and every nth member is selected to be in the sample.
Here, you select samples based on a fixed interval. For example, you might have a list of clients from your gym and want feedback on a new training program. You could use systematic sampling by selecting every 10th person on the list to participate in your survey. You start from a random point, but then the selection is systematic (i.e., following a specific order or system).
Determining Sample Size
Determining the right sample size is a key step in any statistical study. Too small a sample, and you might not capture the whole picture. Too large, and you might be wasting resources. A few main factors go into deciding the sample size. Let's look at these factors.
Population Size: This is the total number of people (or things) you're interested in studying. If you're trying to figure out what people in your city think about a new park, the population size would be the total number of people in your city. Generally, if you have a larger population, you'll need a larger sample to accurately represent everyone's opinions.
Confidence Level: This is a bit like a safety net. When we pick a sample, we know it might not perfectly match the whole population, but we want to be reasonably sure it's close. The confidence level is our level of sureness. If we set a confidence level of 95%, it means that if we were to repeat our study 100 times, we would expect the results to be within our estimated range 95 times out of 100.
Margin of Error: This is an allowance for some amount of error in our results. It's like a buffer around our estimate. For instance, if our survey says 60% of people like the new park, with a 5% margin of error, the actual number could be anywhere between 55% and 65%. The smaller you want this buffer to be, the larger your sample size needs to be.
Population Variance: Think about how different the people in your population are from each other. If you're surveying people's height, there are a lot of variances: some people are short, others are tall, and many are in between. In cases where you expect a lot of variances, you'll need a bigger sample to make sure all these differences are captured.
All these factors can get tricky to balance when deciding on your sample size. The key to determining sample size lies in balancing accuracy and resources. A larger sample can provide more accurate results, but it also requires more time, money, and effort to collect and analyze.
So, there you can have it, armed with this new knowledge of sampling, no more blindly accepting findings. You are now better placed to judge the reliability and validity of the study outcome.