What is Data Virtualisation?
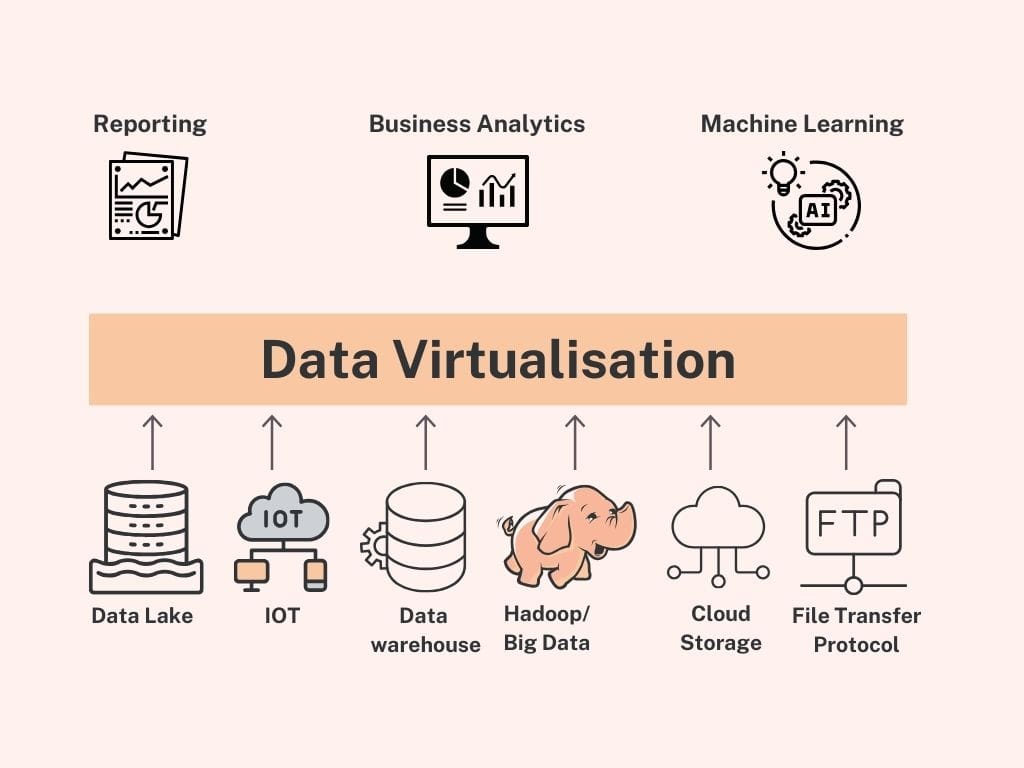
Hi Everyone👋
I've been on a hiatus. I started a new job and have been busy learning the ropes. It's been a while since I felt like a newbie, going from someone who knew most things at work to someone who is learning again. While challenging, it's also made me feel more alive. Sometimes, staying with the same company for too long can make you lose that initial excitement, but joining a new company has brought that buzz back.
So, what have I been up to lately? I've been quieter on social media, focusing more on work and completing the Google Advanced Analytics Professional Certificate. This six-month Google certificate program covers topics like statistics, data visualisation, and common machine learning models like regression, random forest, and Bayesian models. It also teaches different methods to evaluate model performance. I wanted to become a data scientist for quite some time, but this program has confirmed that I don't enjoy it all that much. I'm more fascinated by data engineering work. Data engineering work reminds me of my fond memories of building circuits in physics class in high school. I'm not 100% sure which direction to pursue yet and will have to do more exploration. One of the great things about a career in data is it is incredibly vast and offers numerous paths and opportunities.
In addition to the above, I've also been exploring data virtualisation. I first encountered this term at my previous job when I saw a diagram with various data sources and a banner above them reading "data virtualisation." It didn't seem easy then, so I left it at that. However, the term came up again recently when I was researching a company called Starburst, which specialises in data analytics tools and solutions. This piqued my interest, and I decided to learn more about data virtualisation. So here we are today, discussing data virtualisation.
Here's what we'll cover:
- What is data virtualisation?
- The history of data virtualisation
- How data virtualisation works
- The benefits and challenges of data virtualisation
- What is data virtualisation?
What is Data Virtualisation?
If you work in a corporation, chances are your company has likely already adopted data virtualisation, but you may not know about it.
Data virtualisation is a data management approach that allows users and data professionals to retrieve and manipulate data for analytics and models without knowing the technical details about the data, such as how it is formatted or where it is physically located. So it can give the user a view of the overall data irrespective of where it's stored.
If this sounds abstract, let's consider an analogy. Imagine you want to watch a movie. You might have had to dig through a pile of DVDs in the past. Some DVDs might be at your friend's or your parent's. Today, with platforms like Netflix and Amazon Prime, you can access a vast content library from a single interface. This convenience and efficiency are akin to what data virtualisation offers in data management. Data virtualisation allows organisations to access and manipulate data from multiple sources without needing to move or replicate it, simplifying data handling as much as modern streaming services simplify our entertainment options.
History of Data Virtualisation
Data management has changed a lot over the years. In the past, organisations used physical files and essential databases to store and retrieve information. As the amount of data grew, they needed better ways to handle it. During the 1980s and 1990s, data warehousing became popular. This involved gathering data from various sources in one place for analysis, but it was often expensive and slow.
In the early 2000s, data virtualisation was developed to tackle these problems. It created a virtual layer that lets users access and work with data from different sources without physically moving it. This was a significant change in how organisations managed data. Essential developments included tools like Composite Software (later bought by Cisco) and Denodo, which helped make data virtualisation more common and effective. Over time, data virtualisation has improved, adding features like real-time data integration and support for big data platforms.
How Does Data Virtualisation Work?
Data virtualisation uses a virtual layer to connect and integrate data from various sources, such as databases, applications, and big data platforms. This virtual layer acts like a librarian who knows precisely where to find every book in a vast library, even if the books are spread across different rooms or buildings. When a user requests data, the data virtualisation layer retrieves the necessary information from the relevant sources and presents it in a unified format. This process eliminates the need for data replication and centralisation, streamlining data access and integration.
The Benefits and Challenges of Data Virtualisation
Data virtualisation offers several significant benefits:
Simplified Data Access: Users can access and query data from multiple sources through a single interface, reducing the complexity of data retrieval.
Improved Data Integration: It allows seamless data integration from various sources, enabling comprehensive analytics and reporting.
Enhanced Agility and Flexibility: Organisations can quickly adapt to changing data needs without the lengthy data migration or restructuring process.
Cost Efficiency: Data virtualisation reduces storage costs and minimises the need for extensive IT resources by eliminating data replication and centralisation.
Despite its benefits, data virtualisation also presents some challenges:
Data Governance and Compliance: Ensuring data security, privacy, and regulatory compliance across multiple data sources can be complex. Maintaining consistent data governance policies is challenging as data is spread across various locations and systems. Organisations must implement robust data governance frameworks to address data quality, lineage, and access control. This involves setting clear policies on who can access data under what conditions and ensuring that these policies comply with relevant regulations such as GDPR or HIPAA.
Performance Issues: In some cases, performance may be impacted when large datasets from multiple sources are queried. The virtual layer adds an abstraction that can introduce latency, especially if the underlying data sources are not optimised for quick access.
There we have it. Data virtualisation is a valuable tool for today's data management. Hands down 🙇♀️ to the group who came up with it; it's such an intelligent solution to manage data! Who would have thought the best way to move data is not to move data at all? Integrating all the data sources without moving any data is a great way to simplify access and integration while being cost-effective.