What is the Data Analysis Process?
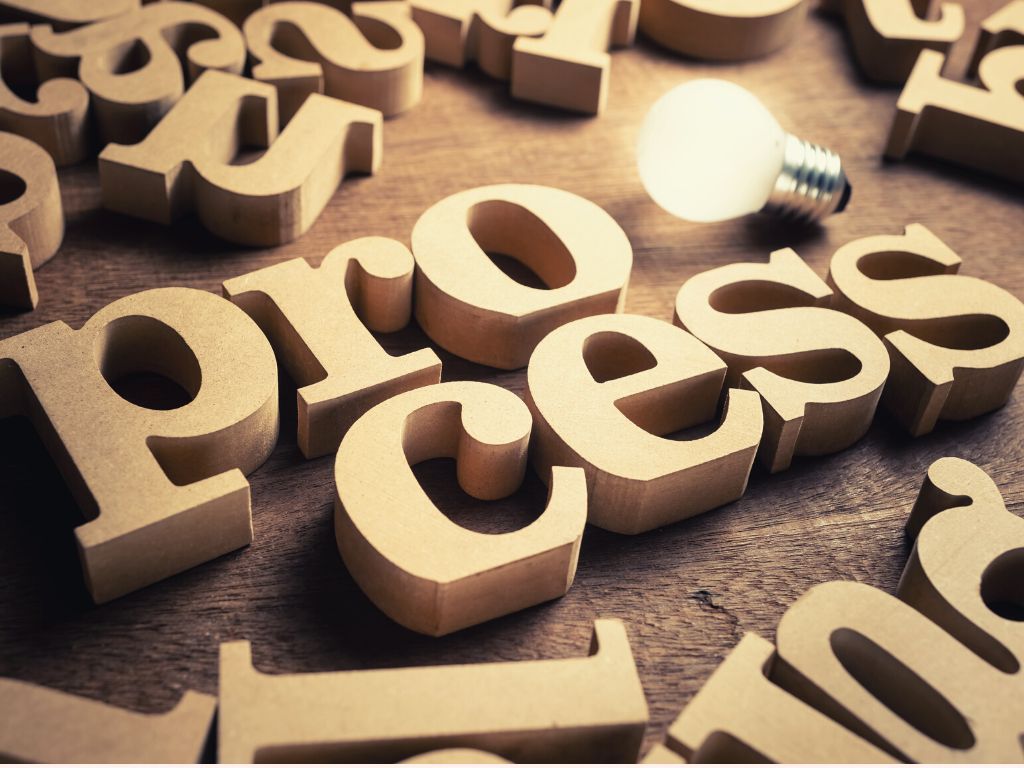
Data analysis plays a role in making decisions more scientific and helping businesses operate more effectively.
It is the process of collecting, cleansing, transforming and modelling to discover insights to inform conclusions and support decision-making.
When we speak about analysing data, we follow a process to reach our goal of extracting meaningful insights. In this blog, we'll explore the process.
The Data Analysis process is an iterative process that contains five core steps. They're outlined in the image below.
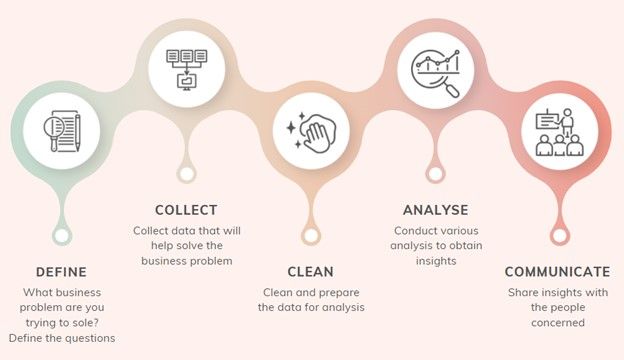
1. Define the Questions
The initial step of the process is determining your objective, i.e., the business problem you're trying to solve. The technical term is the problem statement. It's helpful to frame it as a question. Doing this helps you focus on finding a clear answer.
Apart from defining the objective, it is also essential to think and determine which data sources will best help solve the business problem.
This step is mostly about soft skills, business knowledge and lateral thinking. Business knowledge comes in handy here. You may have a hunch to help you follow.
2. Collect Data
Once you have your problem statement and determine the data sources you need, it's time to gather and collate them. The sources are often varied and range from organisations’ databases to information from websites. All data fit into one of three buckets: first-party, second-party, and third-party data. Let's quickly explore these.
First-party data is the data you or your organisation have directly collected from your customers or audience, e.g. transactional information from the point of sale (POS) system, information from customer relationship (CRM) system, customer satisfaction surveys etc.
Second-party data is the first-party data of other organisations. You may want to use second-party data to augment your analysis.
Third-party data refers to data collected and aggregated from numerous sources by an organisation. The organisations do not directly interact with customers or the business data consumers—E.g. data from non-profits, demographic data etc.
3. Clean Data
Data cleaning is a very time-intensive step; it is widely referenced that 60-80% of data analysis is spent on this step.
However, it is an important step because not all data is good. Using wrong data points can severely impact your results. Additionally, this step helps you realise and understand the nuances of the data.
Common cleaning tasks include removing major errors, purging duplicates and outliers, removing irrelevant data that have no bearing on your intended analysis, fixing typos, layout issues and shaping the data so it's ready for analysis.
Usually, alongside cleaning data, you may want to do a bit of exploratory analysis to help identify initial trends and characteristics that can help refine your hypothesis. It may also result in additional data cleaning or requests for data; hence the iteration mentioned at this blog's beginning.
4. Analyse Data
After all that, we're finally ready for the fun bit- analysing your data! The type of analysis to be carried out depends on the goal. All kinds of data analysis fit into one of the four types: Descriptive, Diagnostic, Predictive and Prescriptive.
Descriptive Analytics answers what happened, Diagnostic Analytics answers why did it happen, Predictive Analytics answers what is likely to happen and,
Prescriptive Analytics answers how it will happen?
Check out my blog on What are the Different Types of Analytics for more details.
5. Communicate Results
The final step is to share your findings with the people concerned. You may have feedback, which results in additional analysis.
It is vital to ensure that you cover everything unambiguously and concisely and that your conclusions are fact-based. Any gaps in the data and insights that might be open to interpretation should be highlighted and flagged. How the results are interpreted and exhibited by analysis can significantly impact the course of a business.
That's it, folks. These are the five primary steps in the data analysis process; it is iterative and underpins every data analyst's work.